Reduce technical barriers and tackle AI implementation challenges with 3D deep-learning technology
Date Section Blog
Summary
A rapidly growing population places pressure on natural resources and food security. Farmers, agronomists and greenhouse owners will need to move beyond traditional farming to meet the increasing demand for sustainable food production. Artificial intelligence (AI) can potentially help increase efficiency, streamline processes and provide employment opportunities. However, AI brings the most value only when it is scaled operationally. Businesses in traditional industries such as agriculture and food are faced with a host of challenges: a lack of technical personnel to develop AI models and AI implementation hurdles including variability of nature, seasonality of data and fleet management of robots. AI vision technologies can help companies scale AI in agricultural robotics by standardising hardware and scaling human intelligence. Robovision offers a self-serving AI-powered platform to facilitate rapid, secured, and efficient development of AI.
About Robovision
Robovision has transitioned from a consultancy company into a software company. With Robovision’s platform, farmers, agronomists and greenhouse owners can leverage our tool to create Artificial Intelligence (AI) models from their own data and without data scientists involved. They can also deploy these AI models on their own greenhouse automated machines or on their agricultural robotics. As one of the fastest-growing tech companies in Belgium, Robovision is a privately held company, with more than 120 engineers and data scientists.
Historically, Robovision started out with consultancy, because automated algorithms were not something we believed in. Our mission back then was to make flexible machines that combine robots with camera acquisition devices. After delivering the first series of machines to the market, there was a high number of requests for building machines that can adapt to different plant types and in different kinds of conditions. As a consultancy company, work was carried out on a project basis and machines were only built to fit a predefined purpose. Our clients, however, expected more flexibility in the products delivered and in our services. This demand helped us envision Robovision today. We have become a product-based company developing an AI-powered platform that can help companies solve their scalability problems.
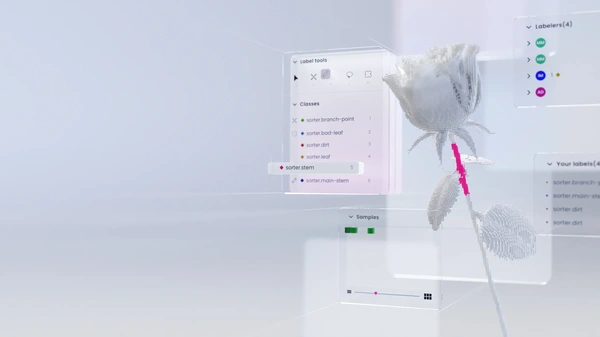
A pioneer in 3D deep learning
In 2015, we already leveraged machine learning and deep learning technology in our product development. Robovision as a company was a pioneer at the time and using our software as a generic algorithm creation tool. Getting more information from real-world visualisations was a major challenge that we tackled. After working with 2D data for a while, we realised that certain products such as tulip bulbs, cauliflowers and carrots are volumetric. 2D AI would be unable to solve complex visual tasks for these products. Unlike 2D data, 3D data is rich in scale and geometry information, thus providing a richer and better environmental understanding for machines.
3D deep learning hence can revolutionise agricultural robotics. Not only we invested in 3D acquisition devices, but our team was also working hard to make sure we could apply this deep learning technology in the field of 3D. Robovision is the first company to bring to the market a new generation of planter machinery. This deep learning-based machine can actually plant, for example, a wide variety of tulips. Beyond this big success, we want to use this technology to solve more challenging use cases, such as 3D automated sorting and plant cutting applications. Rose cutting and phenotyping such as DNA sampling are also some of the advanced applications of 3D deep learning technology. In the coming year, our ambition is to tackle more and more challenges in the food industry with Robovision’s AI platform.
Expanding AI applications across agricultural processes
Food is an important industry that is facing many risks. With an increasing global population and resource scarcity, technological developments such as AI can help make the entire food production process more efficient. By leveraging AI, not only in vertical farms, but also in outdoor robotics, advanced self-serving platforms such as Robovision AI can be used to fight the diversity of nature, and ensure that AI-based machinery and systems can tackle various conditions. For example, we are working on a new product – smart glasses, together with Iris Stick, that are embedded with NVIDIA hardware. This product can actually help the operator in the greenhouse to select ripe tomatoes for instance. Besides 2D and 3D, there is also sensor technology. Hyperspectral imaging will be of extreme importance when combined with 3D deep learning in the vertical farms of the future. This is where companies need to invest in the right technology with the right tools.
How to scale AI in agricultural robotics
Reducing technical barriers
At the beginning of our platform development, we quickly realised that farmers and greenhouse owners faced a major common problem: they are not technically skilled enough to work on an AI project on their own. For many of these algorithmic maintenance workflows, companies will need to have data scientists or highly technical personnel in the team. We embarked on a mission to engineer a platform that is very easy to use for non-technical users. An operator without any IT knowledge could label a couple of images, and after 15 minutes they could deploy the labelled dataset to the machine efficiently.
The typical activities of an operator in an AI project are mainly about labelling. Nature diversity requires expert knowledge to label data and the technical nature of the labelling tasks can affect AI adoption. With Robovision’s AI platform, however, non-technical operators can collaborate with staff agronomists and plant experts to develop AI.
The labelling tool is being used to speed up the process of creating large-scale image datasets. Different kinds of tooling will be required to achieve speed. As mentioned earlier, there is a need for both 2D and 3D data types. We thus enlarged the 2D labelling capability in our platform to include 3D annotation features. This labelling advancement is not only used in agricultural technology (AgTech) but also in other fields like healthcare. To date, Robovision’s AI platform has the most extensive 3D labelling toolset in the world. Some of our labelling tools are even tweaked to meet agricultural needs, such as lasso, connectivity, direction, vector and many more.
Rose cutter is amongst the automated agricultural machines that applied 3D deep learning technology from Robovision. Together with our original equipment manufacturing (OEM) partner, we built a machine that can cut 1,600 roses per hour automatically. This requires 2D cameras, calibration software and annotated data. We offer a tool like AutoCAD that can train the AI model, which understands the plant and points out which side of the branches to cut.
Every farmer is unique and so is every rose type. Farmers or operators will need easy wizards or AutoCAD-like tooling to annotate. They first need to label new data in order to train a new rose type. They can do so easily on Robovision’s AI platform. The whole labelling process is powered up with an NVIDIA GPU embedded into the system. Without depending on any support team, they can develop AI and move it to the real world with full control of the process.
Tackling AI implementation challenges
As the global population is growing, there will be more people to feed. AI technology can support the growth of food production in agriculture in the future. Companies like Robovision can help increase efficiency by leveraging the data derived from AgTech processes. The big question is: why isn’t this yet mainstream? We saw there are three main reasons: the variability of nature, the seasonality of data and fleet management of robots.
Challenge 1: Variability of nature
Food produced in natural conditions is extremely difficult to control due to diverse influences, such as humidity, drought, floods and so forth. This variability of nature thus makes every farmer and every greenhouse unique. To solve this challenge, our Robovision platform offers a simple way to retrain AI models. The operator or a non-technical person can easily look at a couple of plants, label them in 2D or in 3D, then deploy it as a new AI model in only 15 minutes with a click of a button. We have clients with even thousands of deep-learning models developed on our platform. The biggest value we offer is not a single data scientist working inside those companies. Beyond that, companies are creating more jobs as new employees are being hired to operate AI-based machinery. We turn farmers or plant experts into AI operators even without any technical expertise.
Forensics, an integrator of our machine-building partner ISO Group, runs these neural networks with machines that leverage the GPUs of NVIDIA. They retrain 3D deep-learning networks in order to support a new product type, such as tulip bulbs. There is a need to find the right growth vector inside a tulip bulb, in order to let the neural networks understand what the growth direction of that certain tulip type is.
Challenge 2: Seasonality of data
In agriculture, once companies have botched a certain automation implementation, they will need to wait for another season to start over again. This leads to a lot of delay. If a machine has not yet reached perfection, companies will need to wait for another year. How do we cope with this seasonality at Robovision? The answer is synthetic data. When Mark Zuckerberg talks about the metaverse, we think of the greenhouse metaverse. This means we can simulate all kinds of conditions: simulating droughts, plants with small leaf types or even humidity. Synthetic data enables us to be well prepared for this first validation of a new product line or to tweak it to certain conditions. If we can generate metaverse data, simulated data or synthetic data, we will automatically know where the side branches are or how big the leaves are. We have more than 150 NVIDIA GPUs in our data centre to support this synthetic data creation.
Challenge 3: Fleet management of robots
With different installations of robots and drones, companies will need proper fleet management. All of these edge devices will need to be updated with the right AI model. Our Robovision AI platform supports this fleet management need, with full AI lifecycle management. Scaling with computer vision should be a breeze. There is no longer a need for a big team of data scientists. We offer the necessary test automation and DevOps scripts for edge deployments. By leveraging Robovision’s data science knowledge, companies can enable operators or domain experts to work on AI projects, without having an extensive education of AI. Lastly, Robovision brings the state of the art to our platform with the robust 2D and 3D deep neural networks in an intuitive user interface.
Over 600 robots use AI applications created with our platform in more than 40 countries. They are all running with minimum support from Robovision, because the operators, the farmers and the greenhouse owners can use the technology by themselves. Through trial and error, they learn to train a new neural network with the required number of images. The user takes ownership of training 3D deep-learning models, which also enables scalability.
Conclusion
As every farmer is unique, they expect the ultimate robustness and flexibility of their agricultural machines. Machines provided by well-known suppliers such as John Deere should be standardised, because standardisation makes service and maintainability much more manageable. The future of AgTech, from our perspective, is standardised hardware and scaling expert knowledge. With expert knowledge, we meant domain experts or non-technical operators that can use a self-serving and no-code AI platform to annotate complicated plants like roses or tulips and be able to manage these AI workflows by themselves.
The more data you annotate, the better the AI models are. Robovision has access to a large pool of 2D and 3D annotators. If a farmer in Canada for instance wants to train a new tulip type, the data just needs to be made available in the NVIDIA cloud. Annotators from anywhere around the globe can then work on the assigned annotation tasks. They are supervised within the platform in such a way that the training can be done in a breeze. All in all, companies can scale AI operations with an AI platform that leverages the power of 3D deep learning for AgTech.
Quotes
The future of AgTech, from our perspective, is standardised hardware and scaling expert knowledge. With expert knowledge, we meant domain experts or non-technical operators that can use a self-serving and uncomplicated AI platform to annotate complicated plants like roses or tulips and be able to manage these AI workflows by themselves.
Jonathan Berte, CEO of Robovision