Robovision 5.9: The Next Evolution of Data Labeling
Date Section News
Robovision’s 5.9 release introduces Foundation-Assisted Labeling, powered by the Segment Anything Model (SAM). Correctly masking objects in images for datasets is the most time-consuming aspect of any vision AI project. This advancement significantly reduces the time and effort required to create initial datasets for model training and development.
Automating key stages of the labeling process accelerates model creation; at the same time, accuracy is fully maintained
From Grabcut to Predictive Labeling: A History of Progress
In its early days, Robovision introduced Grabcut to simplify the creation of masks for both instance and semantic segmentation. By drawing a bounding box around an object, a mask is generated automatically, but would in many cases still require some manual corrections.
Building on this, predictive labeling allows users to annotate only a portion of the initial dataset. A model is then trained on only the subset to automatically label the remaining data, still with opportunities for refinement as needed. This approach is valuable not only because it accelerates accurate model creation, but because it also provides valuable insights into model performance during early development stages.
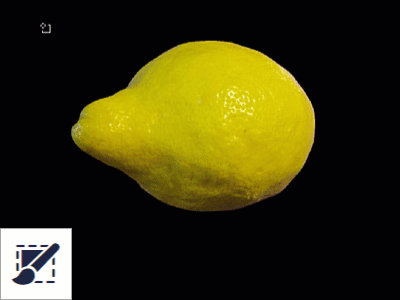
A New Era: Foundation-Assisted Labeling
Robovision continues to build on this groundwork. The 5.9 release introduces the Smart Mask tool (aka. Foundation-Assisted Labeling), another step forward in labeling efficiency. While predictive labeling still requires some manual refinements to masks, this new feature further automates that step.
Powered by the Segment Anything Model (SAM), which excels at precisely delineating object contours, image embeddings are generated prior to labeling. SAM identifies every potential object within an image, producing embeddings that serve as “selectable” masks. The labeler simply clicks on the most relevant masks.
While minor adjustments are sometimes needed, this is significantly reduced compared to Robovision’s other auto-masking tools. This method significantly reduces manual effort and accelerates the time required to reach the predictive labeling threshold.
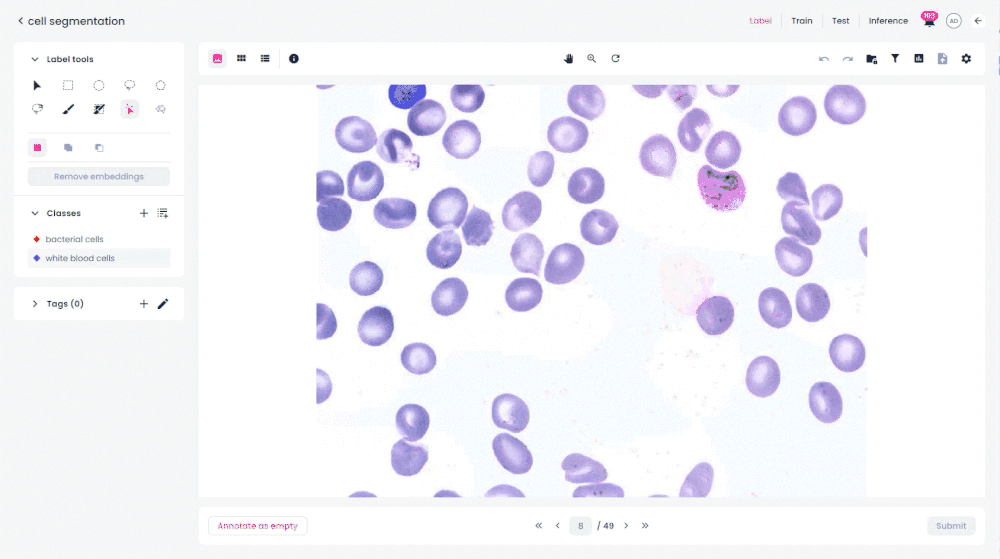
Less manual effort, faster model creation, and improved accuracy.
Looking Ahead: Defect Detection and Few-Shot Learning
The introduction of Foundation-Assisted Labeling marks the first step in Robovision's ongoing journey to improve labeling efficiency. The next step is to extend this capability to defect detection using bounding boxes. This approach will provide pre-annotated bounding boxes with the option to assign classes to the selected areas.
Another planned advancement is Few-Shot Learning, designed to further reduce the need for extensive annotated datasets. With as few as two annotations per class, predictions can be generated across the entire imported set, increasing in accuracy as more annotations are added. This method leverages SAM to focus only on relevant objects, further minimizing manual labeling efforts.
Next, these enhancements will be applied to classification scenarios through sample clustering. By grouping similar images, this technique will enable batch classification, allowing multiple samples to be labeled simultaneously.
Robovision 5.9: What Else is New?
When it comes to developing AI solutions, the journey is always ongoing. Foundation-Assisted Labeling is just one example of how Robovision continues to push the boundaries in vision AI.
The 5.9 release also introduces enhanced grading and picking capabilities designed for easy integration into machinery. This upgrade streamlines product inspection and categorization on conveyor belts—particularly for organic food and plant items that pose challenges for conventional machine vision systems—and communicates with robotic systems for precise removal or packaging.
Inference monitoring will also debut: a real-time “health check” for AI models in production environments, tracking their accuracy and detecting anomalies in performance. It quickly flags issues, ensuring models remain accurate and reliable even as conditions change.