
Computer Vision AI in Pharmaceutical Manufacturing: Defect Detection and Classification
With the swift emergence of technologies like artificial intelligence (AI), robotics, and advanced computing, pharmaceutical manufacturing has seen a huge advancement as it transforms into large-scale production. Implementing these new technologies is never easy, but it is a necessary step when looking to increase output, reduce waste, and more efficiently align with strict safety regulations.
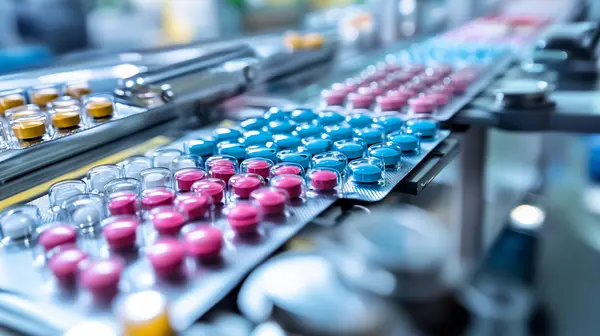
Key Challenges in Advancing Pharma Manufacturing
While Vision AI makes its way into more and more production lines across industries, pharma manufacturing has its own set of challenges.
Meeting Government Safety Regulations and Requirements
Implementing vision AI into any manufacturing line can be a challenging process, especially when working with legacy manufacturing equipment and workflows. But on top of that, government agencies like the FDA need to approve each step of the process according to important safety standards. Implementing new technologies and systems requires a full revalidation of the manufacturing line, which is time-consuming and expensive.
As a result of this challenge, many manufacturers continue operating with lower yields rather than pursuing system advancements, as it ultimately is more efficient because of the cost and level of disruption.
At the same time, the FDA recognizes the value of artificial intelligence in its own official documentation meant to guide the industry’s inspection processes. While there’s no denying the struggle to implement vision AI and get the FDA’s stamp of approval, doing so ensures that strict safety standards can be met more often, resulting in better, safer output.
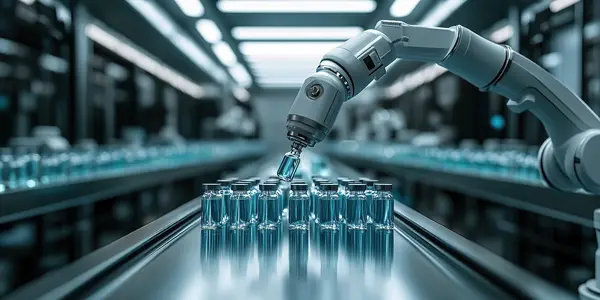
Classification and Management of Rejected Items
The way many pharmaceutical plants manage defective products doesn’t involve sophisticated classification systems that can identify a specific defect in vials, tablets, capsules, packaging, or medical devices. Quality control is simply “good” or “bad” and as long as the Acceptable Quality Level (AQL) remains consistent, then the manufacturing process is considered stable.
Only when the AQL isn’t met, a manual inspection is required. The lack of multiple rejection control points means the inspector must manually search for and identify the root cause before implementing the necessary corrective.
Implementing computer vision AI at this step in production offers a lot of potential. Using deep learning, specific defects are identified and recorded, which means less downtime due to manual inspection. And since the items in question have already been classified as defective, they don’t fall under FDA jurisdiction either, which simplifies the process of implementing computer vision AI into existing systems.
Example Applications of Vision AI in Pharma Quality Control
Pill inspection | Vial counting |
Vial contamination Inspection | Medical device Inspection |
Packaging inspection and identification (bottles, blister packaging) |
Integrate Vision AI and Streamline Production
The Robovision AI platform gives you a fast track to smart solutions by integrating computer vision into your machinery that’s accessible and easy for operators to maintain without AI expertise. This helps enhance quality assurance, optimize automation, and improve safety—without the need for external consultancy or IT services.
Quality Assurance at Scale
Full AI automation that adapts to production growth.
Enhanced Automation
Takes defect detection to the next level: Identify multiple defects across different product types.
Reduce Downtime
Minimize the need for manual root cause analysis and speed up correctives.
Empower Operators
Operators can develop and maintain AI models themselves, without the need for data scientists.
Robovision provides robust platform and Edge integration options through SDKs and APIs, supporting deployment in cloud environments, on-premises setups, or within existing systems.