Wafer Defect Classification: Key Techniques and Industry Standards
Date Section Blog
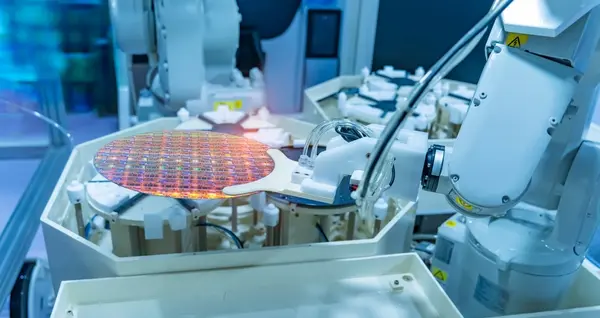
The development of leading-edge chips grows ever more complex. With it comes the increasing need to reduce defects on the wafer edge, bevel, and backside. From chipping to edge contamination—a single flaw can have costly repercussions across multiple processes and multi-chip packages. But with effective defect classification techniques, manufacturers can improve yield, avoid costly breakage, and solve common wafer inspection challenges.
Wafer Production Complexity
There are numerous reasons why it’s so hard to produce high-yielding flawless chips at the 300mm wafer edge. Process variation, wafer uniformity, and multi-layer effects such as film stress all contribute to the challenge. Maintaining across-wafer uniformity is particularly difficult. Leading-edge designs require up to 1,000 process steps per wafer, including patterning, deposition, etching, and plating. Worst case, edge defects are a major culprit behind wafer breakage—disrupting production and leading to high costs.
Accurate detection allows manufacturers to quickly identify manufacturing process faults, adjust, and improve efficiency to minimize scrap rates. However, global demand for chips continues to rise as integrated circuits power smart devices and wireless communication. Meanwhile, lithography processes have advanced to sub-10 nm precision. This progress places higher demands on yield at every production step and intensifies challenges for defect detection techniques in wafer manufacturing. Below are several key defect classification techniques to help manufacturers spot flaws more efficiently and implement corrective measures.
Wafer Map Defect Classification Methods
To eliminate defects, engineers analyze wafer maps to diagnose failures and implement corrective actions to improve process yield. Nowadays, traditional classification still relies heavily on manual inspection. Engineers classify wafer map defects based on their experience—which requires additional labor costs and mostly depends on subjective judgment. Manual defect detection remains common but is prone to errors. Inspectors struggle with efficiency and accuracy, making it difficult to meet the precision demands of modern semiconductor production. Identifying defects early requires a systematic approach that reduces reliance on subjective human judgment.
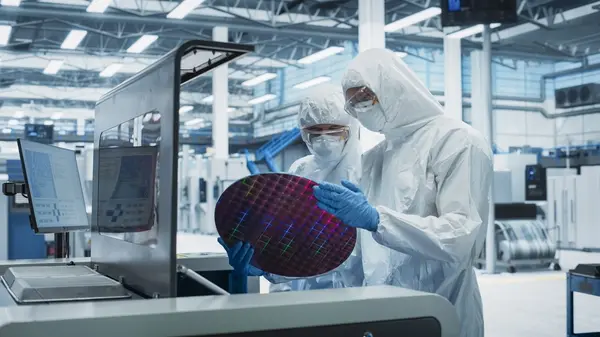
Wafer Surface Defect Detection Based on Image Signal Processing
Image signal processing converts image signals into digital signals, which are processed to achieve image transformation, enhancement, and detection. Wafer inspection techniques—such as spatial filtering, template matching, and wavelet transform—improve detection capabilities.
Wavelet Transform
Wavelet transform (WT) is a signal time-frequency analysis and processing technology. It works by decomposing the image signal into different frequency subbands through a filter to perform wavelet decomposition. Statistical analysis is then applied to detect anomalies. By calculating the mean, standard deviation, or other wavelet measures, it analyzes each coefficient. WT excels at boundary processing and multi-scale edge detection, making it effective at detecting fingerprints and stains. But research shows this method is not as accurate when inspecting sharp-edged micro-cracks: limiting its effectiveness for all defect types.
Spatial Filtering
This mature image enhancement technique is realized by direct spatial convolution application to the gray value. The main role in image processing is image denoising, which is divided into smoothing and sharpening filters. Often, however, spatial filter performance is highly parameter-dependent—making their values difficult to choose.
Template Matching
Template matching detection is achieved by calculating the similarity between the template image and the image under test. This technique detects the difference area between the image under test and the template image.
Neural Networks and Vision AI
The emergence of computer vision-based detection methods, especially neural networks, addresses the limitations of data preprocessing. Neural networks have transformed defect detection by improving efficiency, accuracy, and objectivity. Their low cost and strong objectivity have resulted in rapid development and wide application in wafer defect detection. Nowadays, convolutional neural networks are a reliable method for image classification. They use convolution computation to extract features of different dimensions from an image and outperform human inspectors in recognition tasks.
The Data Issue
However, neural networks have several issues. They require both large amounts of training data plus a large number of parameters and calculations. In addition, the problem of insufficient data grows more serious for wafer map defect classification. Not only does the wafer map imply commercially confidential information not easily disclosed by companies: the cost of data labeling is very high, so data with labels become scarce.
Tools like Robovision’s Purity Loop overcome this problem. Addressing both the subjectivity and objectivity of annotated dataset quality, the system makes it easier to handle large data volumes as production scales. The Purity Loop maintains consistent labeling across multiple teams, sites, or production lines without compromising dataset quality.
Future-Proofing Production with Accurate Wafer Defect Classification
Wafer defect detection plays a critical role in semiconductor manufacturing. Early methods rely on image processing techniques such as wavelet transforms and spatial filtering. While effective, these approaches face limitations. Machine learning however has enabled the development of robust solutions, and deep learning has further advanced defect classification with powerful feature extraction. As integrated circuit manufacturing moves toward 4 nm technology and beyond, surface defect complexity will continue to rise. As an industry standard, ensuring model reliability and robustness remains essential for high-quality production.