AI Strategy Implementation: Turning Potential into Reality
Date Section Blog
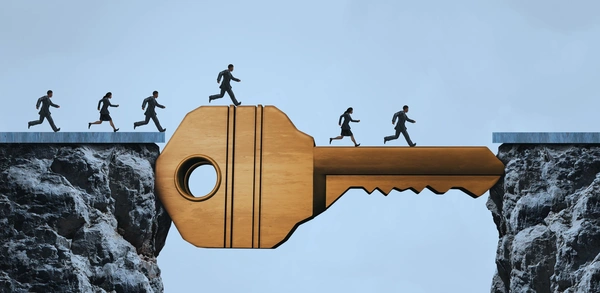
In the fast-paced landscape of artificial intelligence, a perception developed that immediate adoption is necessary to avoid falling behind. The thing is, successful AI integration is not about keeping pace, but about thoughtful, strategic adoption.
Implementing computer vision AI is a complex journey with significant challenges, though it is important to view these as opportunities for growth and innovation rather than insurmountable obstacles. Combining this mindset with effective AI strategy consulting can turn technological hurdles into advancements with impact, and drive business growth and innovation.
From Experimentation to Execution: The Proof-of-Concept Trap
AI solutions often excel in controlled lab settings and polished proof-of-concept (PoC) demonstrations but struggle to deliver in the unpredictable reality of actual operations. PoCs are crafted to impress—showcasing the technology's potential in idealized environments—but PoCs often sidestep the complexities of full-scale deployment.
Real-world implementation demands addressing factors like data variability, system scalability, and integration with existing workflows and infrastructure—issues far removed from the controlled conditions of a PoC.
Understanding these obstacles is critical to bridging the gap between vision AI's promise and its practical benefits. Identifying these challenges early on aligns expectations and jumpstarts the process of finding solutions to smooth AI implementation in operational environments.
Common Hurdles in AI Implementation
Infrastructure Constraints
AI systems require robust, enterprise-grade infrastructure (such as high-performance servers, scalable cloud storage, and powerful GPUs) to handle the volume and complexity of real-world data. Without adequate IT resources, performance issues can arise, making it difficult to scale AI solutions effectively for production environments.
Data Quality and Adaptability
Computer vision AI can struggle in production due to inconsistent, noisy, or biased image data. Unlike controlled PoC environments, actual data is unpredictable—models need to adapt to diverse conditions and handle edge cases to remain effective over time.
Integration Headaches
Implementing AI in production often requires connecting with existing systems and databases. Such complex integrations can create significant roadblocks, particularly in industries reliant on outdated or legacy technologies.
Lack of Operational Expertise
Developing AI models is just the beginning! Operationalizing AI requires ongoing support, including continuous monitoring, retraining, and updating over time. A significant number of organizations lack the internal expertise needed to maintain vision AI systems over time.
Cost and Scalability Concerns
Scaling AI to full production can involve considerable investment in infrastructure, maintenance, and continuous optimization. The long-term financial commitment may seem too large, especially when the return on investment can be far off.
Change Management Resistance
Without transparency, training, and engagement, gaining employee trust and adoption is difficult. If employees resist change, the effectiveness of AI implementation could be undermined.
How to Execute a Successful AI Strategy: Key Considerations
To implement AI successfully in production, it's crucial to first ensure the necessary AI expertise is available. Partnering with experienced teams and investing in your own team’s capabilities paves the way to long-term success.
What’s Involved:
- Partner with experienced AI teams: Robovision has a large team of skilled data scientists, vision engineers, solution delivery teams, and partner managers to realize successful AI deployments and long-term optimization.
- Invest in training: Ensure the internal team has the skills to manage, maintain, and optimize AI models continuously. This includes monitoring performance and retraining models as needed.
- Leverage ongoing support: Establish long-term relationships with AI experts to support troubleshooting, system upgrades, and any performance improvements throughout the lifecycle of the AI system.
Building operational expertise is an ongoing process. It's important to bring in the right support from the start, but maintaining and improving your team's skills and system capabilities are key to long-term success.
Careful financial assessment and strategic planning help balance initial investment with sustainable growth. Start small and scale gradually to demonstrate value early while minimizing risks.
What’s Involved:
- Conduct a cost assessment: Evaluate upfront and long-term costs, including infrastructure, maintenance, and training.
- Start with a pilot project: Develop a small-scale project that demonstrates tangible benefits and builds momentum for further investment.
- Leverage cloud services: Use cloud platforms like AWS, Azure, or GCloud for scalable solutions that minimize upfront infrastructure costs.
A gradual, iterative approach to scaling AI can make the process more manageable. Piloting smaller projects typically takes a few months and provides valuable insights for broader implementations.
To implement AI successfully, the underlying IT infrastructure must be able to support the demands of scaling AI solutions. It’s not just about having strong servers; it’s about ensuring the entire system—hardware, software, and network—can handle the large volumes of data and processing power required.
What’s Involved:
- Assess current systems: Evaluate servers, storage, and network capacity to identify gaps in meeting AI processing and scalability requirements. It might be necessary to upgrade your setup to ensure smooth execution.
- Benchmark performance: Regularly test system performance under different workloads (multiple models running at once, processing large datasets, unexpected spikes in workload) to find and resolve possible bottlenecks. Robovision is benchmarked weekly.
- Explore cloud options: Consider cloud-based platforms for more flexibility and on-demand scaling at reduced costs.
Once the infrastructure setup is suitable, test AI workloads in smaller environments to pinpoint any limitations before scaling. This process typically takes weeks to months, depending on whether upgrades are needed, the complexity of the infrastructure, and scalability needs.
High-quality, diverse, and accessible data is the backbone of successful AI implementation. Ensuring data is reliable, representative, and consistently available is critical to maintaining model performance in real-world environments.
What’s Involved:
- Implement data checks: Use automated tools to detect issues like missing values, duplicates, or outliers. Develop workflows to standardize and validate data formats automatically to maintain accuracy and save time.
- Train with diverse datasets: Incorporate data variability to reflect real-world conditions (like different lighting, camera angles, or backgrounds), improving the model’s ability to handle noisy or incomplete inputs.
- Monitor data drift: Set up systems to track changes in data patterns and retrain models periodically to prevent performance degradation.
- Human-in-the-loop (HITL) systems: Involve human oversight to identify and correct model errors, using production data to refine algorithms.
Part of Robovision’s process is to practice such comprehensive data practices to ensure models perform reliably in operational environments. While implementing these systems may take time and testing, the effort pays off by reducing errors and maintaining consistent AI performance in the long run.
Building adaptable AI models from the start ensures they can evolve alongside changing conditions in real-world operations. Using techniques like transfer learning and continuous learning allows models to respond to new environments and edge cases as they arise.
What’s Involved:
- Use transfer learning: Apply pre-trained models to new tasks to save time and resources. Fine-tune models with smaller, domain-specific datasets to quickly adapt to new contexts without starting from scratch.
- Incorporate active learning: Continuously retrain models with fresh, labeled data (for example, if a new defect emerges) to improve them over time and keep them aligned with current conditions.
- Test in small environments: Before full deployment, validate models in smaller production settings to identify issues and adjust performance before scaling.
This process ensures models remain aligned with current conditions and should be maintained consistently, especially during the early stages of AI deployment.
Once the infrastructure, data, and models are aligned, focus on integrating AI into existing systems. Collaborate with system integration partners or in-house IT teams to address any challenges.
What’s Involved:
- Assess compatibility: Review existing systems to identify potential bottlenecks or incompatibilities.
- Develop a phased deployment plan: Robovision works closely with IT teams or external integration partners to create a deployment plan, allowing for incremental testing, validation, and issue resolution at each step.
- Test and validate: Run comprehensive tests at each phase to ensure minimal disruptions.
Integration is an ongoing process that should continue as your system evolves. After deployment, it’s important to regularly monitor the AI solution and revisit integration strategies as new features or models are added to maintain compatibility.
Successfully integrating AI isn’t just about systems and data—it’s also about people. Promoting AI literacy will demystify the technology, build trust, and empower employees to see AI as a tool that complements their roles, reducing skepticism and misconceptions.
What’s Involved:
- Communicate the value of AI: Clearly explain how AI supports efficiency, decision-making, and employee success.
- Train and empower employees: Provide dedicated learning time (workshops or seminars, for example) to develop the skills employees need to confidently work alongside AI tools.
- Engage stakeholders: Involve team leaders, key decision-makers, and end-users early in the process to build trust and address concerns proactively.
Fostering user adoption is an ongoing effort that benefits from consistent communication and support. Building a culture that embraces AI will make implementation smoother.
Operationalizing AI: A Collaborative Effort
AI implementation requires both technical expertise and a focus on people. Lots of time and consideration go into developing a tangible AI strategy and it’s important to view implementation as a long-term investment.
Partnering with AI service providers accelerates the process as they provide the technical expertise and strategies needed to navigate complex operational environments. Embracing a collaborative approach, AI’s promise can easily become a reality that effectively drives lasting business impact.
Considering AI automation for industrial operations? Our whitepaper helps you evaluate the best approach for your situation.
