Burr Detection: Reducing Defects in Lithium Battery Cells
Date Section Blog
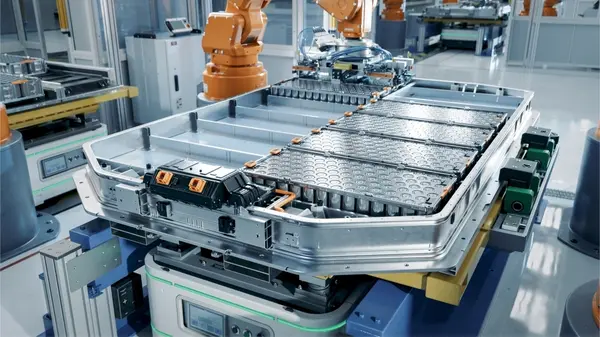
Burrs are tiny metal protrusions at battery electrode edges that cause short circuits and safety risks. Traditional detection methods have struggled to keep pace—but AI-powered inspection is changing the game. Pairing intelligent defect recognition with high-speed adaptive auto-focus cameras, manufacturers can detect micron-scale flaws with unmatched speed and accuracy. The result is safer, more reliable batteries with longer lifespans that set a new standard in quality control.
What Are Burrs on Battery Electrodes?
When electrodes are cut to produce lithium batteries (LIBs) of different dimensions, burrs tend to form in two key areas: the aluminum plate and the welding point of the positive tab. These unwanted metallic protrusions can puncture the separator, causing direct contact between the positive (cathode) and negative (anode) poles. Thermal runaway and short circuits can follow, leading to degraded performance, LIB failure, and—in worst cases—fire or explosion. Research shows even the smallest of burrs can weaken safety.

Quality Control Impact: Tiny Burrs, Big Problems.
To avoid these issues, thorough inspection is needed. However, eliminating burr generation during the slitting and electrode shaping process is difficult due to misalignment or degradation of tools. Despite continuous improvements, the industry still lacks verified and effective detection methods. Burrs are extremely small—often only a few microns in size—making them virtually invisible to the naked eye. Their irregular distribution and unpredictable formation further complicate detection. Quality control and visual inspection of LIB components must be performed at critical production stages, to identify defects before they compromise safety and performance.

Burr size analysis is also essential to assess the risk of battery system damage. Section 5.3.6.2 of the IEEE 1625 standard provides burr measurement guidelines, recommending a comparison of burr size against the separator’s thickness tolerance limit for reliability and safety.
Inline Inspection Methods for Burr Detection and Analysis
Burr detection traditionally relies on offline sampling inspection with electron microscopes. However, this approach is unable to effectively control product quality. To maintain the highest standards, detection and analysis must be efficient and reliable. And this is where inline inspection solutions come in, playing a vital role in quality control by identifying defects before they lead to larger issues.
Microscopy Solutions: Key Considerations
Inline burr inspection often uses optical microscopy to examine the edges and sides of electrodes. The microscope must deliver on speed, efficiency, reliability, and precision—with performance requirements depending on one fundamental factor: burr size. The smaller the burrs, the more precise the detection system must be. Other considerations include illumination and contrast methods, resolution, magnification, image capture speed and storage, ease of data access, and measurement tools. Each factor helps guarantee accurate and consistent detection and analysis results.
Lighting the Way for Burr Detection
Burr size also dictates the optimal lighting method. Microscopes can be used with a ring light, near-vertical, diffuse, and polarized illumination to enhance detection accuracy and reliability. Polarized light improves contrast against the background when observing electrode sedges, while diffuse illumination reduces glare from metallic burrs, simplifying detection. Additionally, the inspection microscope should offer easy access to stored images and burr measurement data for analysis.
Space constraints and investment costs add to inspection challenges—especially when identifying micron-level burrs in high-speed production conditions. Most machine vision systems use off-the-shelf light samples chosen through trial and error. These systems often fail to match the burr surface properties. Stable and precise detection requires a more tailored approach to illumination and imaging technology.
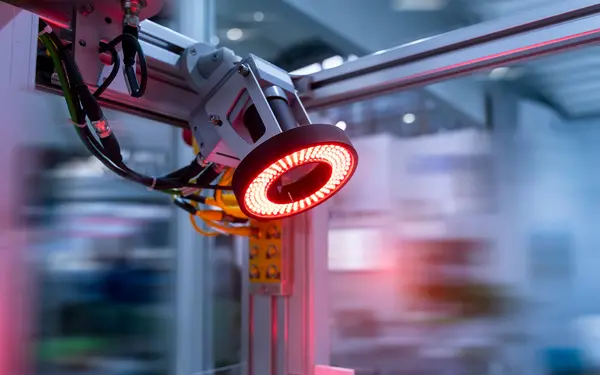
Visual Inspection Powered by AI: Boosting Quality Control Precision
Adaptive high-speed auto-focus systems provide a breakthrough solution for burr detection. By enhancing depth of field, they maintain stable, sharp imaging within the tiniest of vibration ranges in electrode sheet materials—allowing full inspection of edge burrs. However, clear visibility solves only part of the equation. An effective inspection system must also distinguish defects with precision.
Interfering defects such as debris and wavy edges are common during electrode sheet slitting and production. To accomplish zero missed burrs and reduce the false detection rate, strict parameters must be set. The solution lies in accurate classification and filtering of burrs (and interfering defects), which helps prevent material waste and maintain high inspection reliability. Where manual methods prove time-consuming and error-prone, AI automates and standardizes the process of labeling large datasets. By adopting workflows such as predictive labeling, customers can create vision AI models with limited data, deploy them within the production environment, and incrementally improve quality by capturing and labeling new data efficiently.
Battery Electrode Complexity Solved by AI
Burrs vary in length, adding another layer of detection complexity. AI plays a crucial role in solving this challenge. Traditional algorithms combined with AI perform initial defect screening, followed by deep learning AI algorithms such as Convolutional Neural Networks (CNNs) for object detection, defect recognition, and classification. Trained on vast datasets, these algorithms excel in identifying defects such as scratches, cracks, and misalignments with exceptional precision. This allows users to recognize and manage defect labels captured from different cameras across multiple production lines and sites.
While this dual-layer process minimizes false positives and missed detections, AI’s true strength lies in its adaptability. As new defect types emerge, models can quickly be retrained, ensuring continuous improvement and adaptability. The more data the model processes, the better it gets at spotting new defects. This ability to learn and refine makes AI-driven inspection systems a game-changer in battery manufacturing.
Burr Detection: A New Standard in Battery Quality Control
As the EV market grows, so does the demand for high-quality LIBs. AI-powered visual inspection combined with high-speed adaptive auto-focus cameras offers fast, accurate identification of defects, maintaining battery performance standards while reducing failure risks. The potential is vast, with the impact of accurate detection extending far beyond safety. With the right technology, manufacturers can cut material waste, optimize production, and set a new standard in battery quality control.