AI in Agriculture: Sustainable Farming with Cutting-Edge Tech
Date Section Blog

As AI evolves alongside global warming, a paradox emerges: Often viewed as artificial, AI and tech are uncovering the hidden complexities of natural ecological systems. It reveals patterns, dependencies, and vulnerabilities that have long escaped human observation.
The effects of industrialization on the environment are undeniable, as is the skepticism surrounding AI’s rapid adoption. Yet AI is more than a tool of “progress”—artificial intelligence in agriculture has the potential to reshape how industries engage with the natural world, offering interventions with a precision that mirrors nature’s own adaptations.
Nowhere is this clearer than in agriculture, where AI is redefining the future of ecological intelligence. Advances in 3D imaging, synthetic data, and precision robotics are driving some of the most significant technological breakthroughs in the field.
3D Imaging Techniques: The Foundation of AgTech Innovation
Informing AI with advanced 3D imaging is transforming the way agricultural ecosystems are understood, monitored, and maintained.
Providing detailed insights into plant structures, growth patterns, and environmental interactions, these vision technologies look below the surface, aiding precision farming, sustainable land management, and long-term food security.
LiDAR and Stereo Vision: Mapping Agriculture in 3D
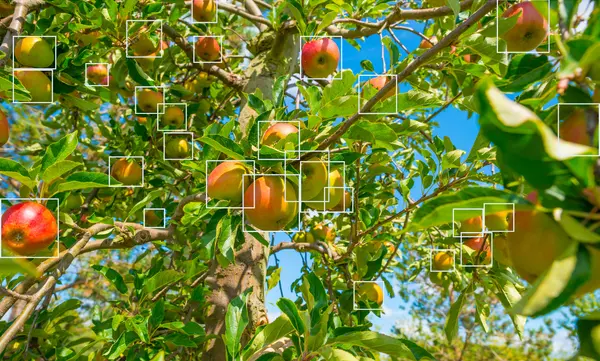
Unlike traditional imaging, LiDAR (Light Detection and Ranging) and stereo vision create precise depth maps, enabling accurate plant modeling, canopy analysis, and robotic automation. Stereo vision mimics human depth perception using multiple camera angles, while LiDAR measures distances with laser pulses, creating real-time 3D modeling of crops and terrain.
Some real-life applications of these vision technologies include:
- Orchard Navigation Robots: A LiDAR-based mapping system uses a 3D-to-2D grid algorithm to detect tree trunk positions and calculate precise navigation points for robots performing tasks like spraying, fertilization, and harvesting in an apple orchard.
- Stereo Vision for Apple Sizing: Researchers developed a stereo vision system with synchronized cameras and LED strobe lighting to measure apple size in orchards on a moving platform, enhancing yield estimation and harvest planning.
Even in orchards with dense canopies and irregular layouts, these technologies improve predictive modeling and farm automation. LiDAR penetrates foliage for accurate structural assessments, while stereo vision reconstructs occluded objects from multiple viewpoints.
3D Multispectral and Hyperspectral Imaging
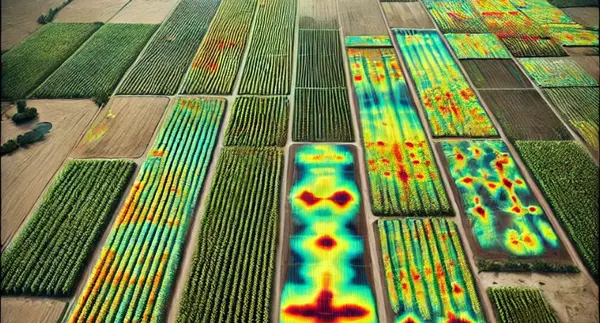
Hyperspectral and multispectral imaging capture wavelengths beyond visible light, revealing biochemical and structural variations in plants. AI processes these spectral datasets into detailed 3D models, detecting changes in crop health, nutrient levels, and environmental stressors that would otherwise go unnoticed.
- Hyperspectral Disease Detection: A study on Fusarium wilt in strawberries found that AI models trained with hyperspectral images could detect disease severity before visible symptoms appeared, allowing for earlier intervention.
- Multispectral Seed Quality Assessment: Researchers used multispectral imaging to evaluate seed viability and vigor by analyzing spectral data to detect internal and external seed traits, supporting breeding and precision planting.
- Aerial Crop Monitoring: Researchers have applied aerial hyperspectral imagery combined with deep learning algorithms to crops like wheat, allowing for high-throughput yield predictions across large agricultural areas.
Early stress detection, seed optimization, and improved yield forecasting reduce water, fertilizer, and pesticide use by allowing for more precise applications based on real-time plant needs. These technologies improve efficiency, but they also pave the way for sustainable agriculture and sustainable farming on a global scale.
Synthetic Data Generation: Bridging the AI Training Gap
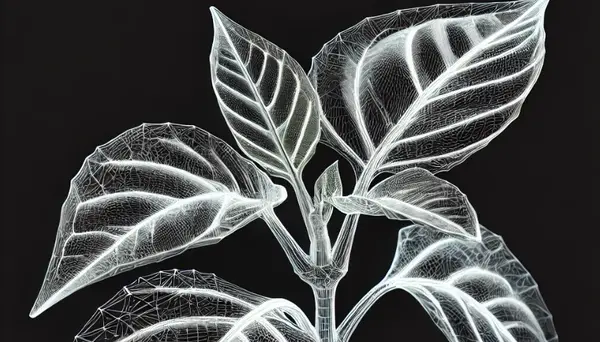
AI models for agriculture require vast, high-quality datasets to function effectively. Real-world data collection is often expensive, time-consuming, and subject to environmental limitations.
To address this, synthetic data generation is a powerful alternative to manual data collection. AI can train on physically accurate, photorealistic datasets without the need for thousands of manually gathered and labeled images.
The ability to train AI models using synthetic data expands research capabilities and accelerates AI deployment in precision farming. Training models to simulate plant behavior, climate effects, and disease progression can improve crop classification, disease identification, and the automation of farming equipment.
Some examples of synthetic data projects in agriculture include:
- Synthetic Data Generation for Plant Phenotyping: Researchers created the Synavis framework, which links functional-structural plant models with Unreal Engine to generate synthetic datasets for plant phenotyping. Simulating environmental conditions like wind and leaf diseases enhance deep learning applications for plant trait analysis and model parameter extraction.
- Predictive 3D Digital Twins: Researchers at AIIRA are developing virtual simulations that mirror the life cycles of biological systems. The aim is to help overcome data limitations by generating diverse training datasets and improving predictions for climate resilience, pest management, and precision agriculture.
Agricultural Robotics: Tackling Delicacy and Versatility
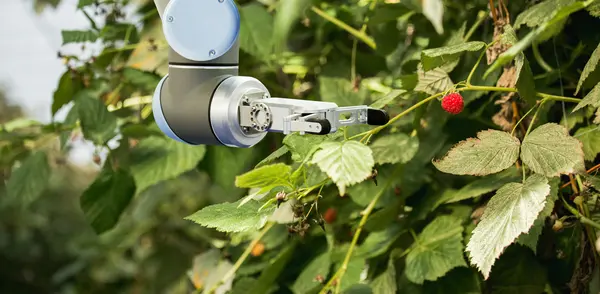
Even with cutting-edge 3D imaging creating perfect datasets, agriculture robots must precisely locate and handle crops for automation tasks to benefit. AI and different combinations of 3D imaging make it possible to detect, localize, and predict fruit movement before picking.
Advances in robotic gripping are also making strides. Traditionally, picking mechanisms are developed for a specific fruit type, but new innovations in soft-grasping technologies show more versatility.
Some innovations in robotic gripping for delicate fruits include:
- Electro-Adhesion Technology: OmniGrasp enables robots to securely yet gently pick soft fruits like strawberries and tomatoes, adjusting grip strength dynamically for differently shaped objects.
- Biomimetic Soft-Grasp Robotics: A rose-inspired gripper being developed in Japan adapts its shape to grip many types of fruit, minimizing pressure while maintaining a secure hold, reducing the risk of bruising or damage.
- 3D-Printed Small Fruit Grippers: Designed for harvesting small to medium-sized fruits, such as blueberries, this cost-effective, compact, and easily replicable soft gripper uses 3D printing with flexible thermoplastic elastomer filament.
The Future of AI in Agriculture: Deeper Observation, Smarter Action
AI is no longer just a tool for analyzing agricultural systems—it is actively shaping them. With 3D imaging, synthetic data, and precision robotics, farming is becoming more adaptive, efficient, and resilient. These technologies are moving agriculture beyond passive observation, enabling AI-driven interventions that enhance productivity and sustainability.
As they continue to evolve, new possibilities emerge. More precise resource use. Earlier disease detection. Smarter automation. By bridging the gap between digital intelligence and ecological complexity, AI is redefining how agriculture adapts to a changing world.