87% of AI projects fail to scale. The other 13% aim for collaborative intelligence
Date Section Blog
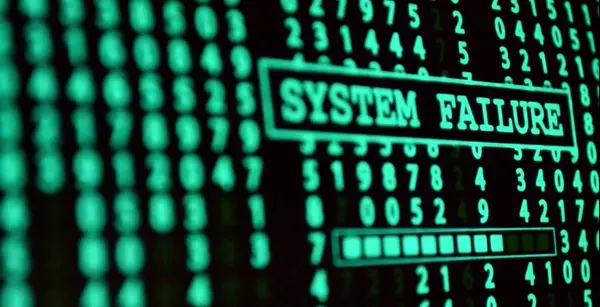
Fact: just one out of every 10 AI projects actually make it into production. The number one reason why they fail is because simply throwing money at an AI won’t do. Other reasons include data that isn’t worth two dimes or the fact that data scientists aren’t used to sharing their expertise. Here are the three main roadblocks AI stumbles upon—and the solution not to fall into the trap yourself. Spoiler: creating AI applications on a collaborative platform will boost your chances of success and finally scale your technology.
Have you ever seen the Monkey Business Illusion video? It shows six people passing around a ball. As a viewer (or test subject, as this is really a psychology test), your task is to count how many times the ball goes back and forth. Go ahead, watch it. We’ll bet you missed the gorilla walking by, or the backdrop curtain changing colour. Don’t fret it, you’re only human. You make mistakes.
But what about a waste plant operator mistaking an explosive gas bottle for common rubbish? Or an oncologist giving your MRI a low-risk evaluation when in fact it should have been instant surgery?
Algorithms are simply better at tirelessly spotting gas bottles than we are. Then again, humans are better at interpreting situations and deciding on the next steps. Combine man and machine, and you’ve got yourself a winning team. So why does 90% of AI stay in the lab, never to get past the conceptual phase?
Reason #1: the data won’t do
The company responsible for waterways and roads in The Netherlands needed to find out why pedestrians repeatedly had accidents on drawbridges. They set up cameras to monitor the incidents, then trawled the data looking for answers.
They found out the AI system responsible for opening and closing the bridge had based its decisions on a single, changing pixel in the image it had been fed: the traffic light. If it went red, the bridge would close. When green, the bridge would open, regardless of any people still being on it. A human eye would spot the error in a heartbeat, but an AI needs training by a patient teacher, who understands that learning is a process and mistakes do happen. In other words: human interpretation is the driving force.
Reason #2: There’s little attention for maintenance
In the first wave of AI, data scientists were the heroes. They had the enormous mission to write algorithms and make them work. In the best case, their work left the concept stage and went live.
These days, data scientists are wanted everywhere, moving from one project to the next. They write the code and off they go, on to the next challenge.
This leaves a lot of gaps in the handover to other engineers or operators, who become responsible for keeping the machine spinning, as well as retraining it to recognize new products or defects.
If these engineers get stuck, their only option is to call the genius data wizard who set up the AI and knows the system inside out—but is by now knee-deep in another project. Not ideal if you’re looking for a quick fix, let alone a solution that scales.
Reason #3: Data scientists are solists
Many companies operate like silos.
Engineers have their own floor, data scientists are freelance and hardly ever come in, and operators are in another building, even.
Here’s the crux, though: if you want your AI to really take off and accelerate your business, you’ll need all of them to work together. A data scientist cannot write a production-grade algorithm without the input of an experienced operator. And the engineer cannot keep the engines running if he hasn’t followed what the data scientists have been up to or where the operators want to take their new tool next. If knowledge remains lodged in silos, AI won’t be the solution, unless it becomes the way to break down the barriers between departments.
It should be a conversation, really
The hattrick to tackle all these issues in one go is a collaborative platform. One in which operators with boots on the ground can train and retrain the AI. One in which they can do so through a user-friendly interface—the same one used by data scientists, in fact, and lets the latter talk in code while operators get a screen with straightforward actions.
What a collaborative platform does, is harness the knowledge of all stakeholders so they can do what they do best. It turns working with AI into a team sport. It allows both ends of the chain to talk to each other and actually understand what the other is saying, strengthening the bond between human and artificial intelligence. It shifts the bulk of the work from the data scientist to the operator, ensuring a proper handover. To boot, it gives operators the autonomy to address any hiccups further down the road.
Rather than operators, they’ll become AI teachers, training their model and watching it grow. Talk about keeping employees happy and machines productive!