The Robovision Purity Loop
Date Section Blog
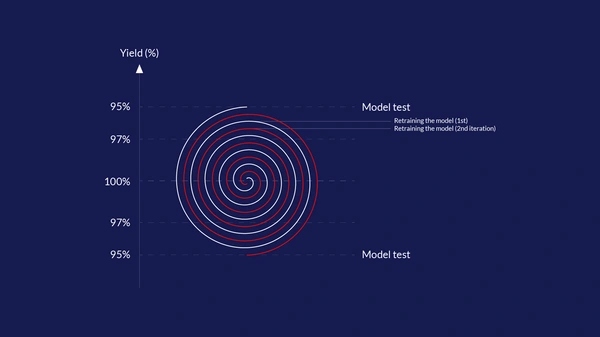
How AI becomes more accurate than the humans who trained it
Pure results need pure data. Or, as the Robovision data scientists eloquently put it: garbage in, garbage out. AI-powered quality control cannot be expected to be 99.95% accurate if your data labeling was only 85% accurate.
Here comes the trade-off. Too many false positives mean unnecessary waste (aka cost). Too many unflagged errors result in poor quality, costing even more. Imagine what could happen if an unflagged defective chip ends up in a car autopilot. Or think of an undetected piece of metal ending up in a baby’s bottle.
Enter the challenge: labeling. A human job that needs robot accuracy because like it or not, every now and then, humans make mistakes. To solve the purity challenge, errors must be detected and then fixed. The system must also be retrained to become more accurate.
Enter the Purity Loop by Robovision.
The Purity Loop is a continuous feedback system designed to refine the accuracy of training data. It pushes AI to be on its best behavior which is necessary for AI systems that tackle complex, high-stakes tasks. Think of semiconductor defect classification or foreign object detection in food production.
The Solution: A Self-Correcting System
At the heart of the Purity Loop lies what Robovision calls the Confusion Matrix. With one click, it highlights inconsistencies between human-labeled data and automated classifications. Discrepancies between human and AI labeling are pinpointed and samples where errors are most likely to occur are flagged.
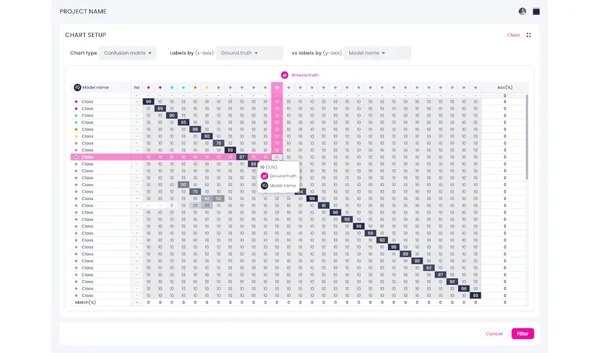
Each flagged instance goes to a Label Center to be re-examined (and re-labeled if needed). This cycle—review, correct, re-train—operates like a self-improving loop that gets “purer” with each iteration, as the name Purity Loop suggests. The result is an AI model that not only learns from humans but also improves human limitations. Step by step, users can move closer to near-perfect classification accuracy.
Since the Robovision AI Platform can be deployed anywhere in a production environment, Robovision 5.7 enables manufacturers to trust their data at every stage. By achieving this level of labeling purity, the AI system can distinguish good wafers from defective ones.
With fewer false positives in defect detection, yield improves—leading to lower costs and increased efficiency in semiconductor production. But the implications go far beyond quality control.
Beyond Manufacturing: Implications for the Future of AI
The Purity Loop can be used in manufacturing, but its potential is nearly limitless. Any field that relies on machine learning can benefit from a built-in feedback loop to refine data accuracy (this includes medical imaging and autonomous vehicles). In an era of data-driven decision-making that affects people's lives from banking to insurance to healthcare, it is reassuring to know that data can be trusted.